

With steep origin kernel estimation, bandwidth selection is replaced by exponent selection and data-based selection is possible. The new estimates are shown to have limit normal distributions, and formulae for the asymptotic bias and variance are derived.
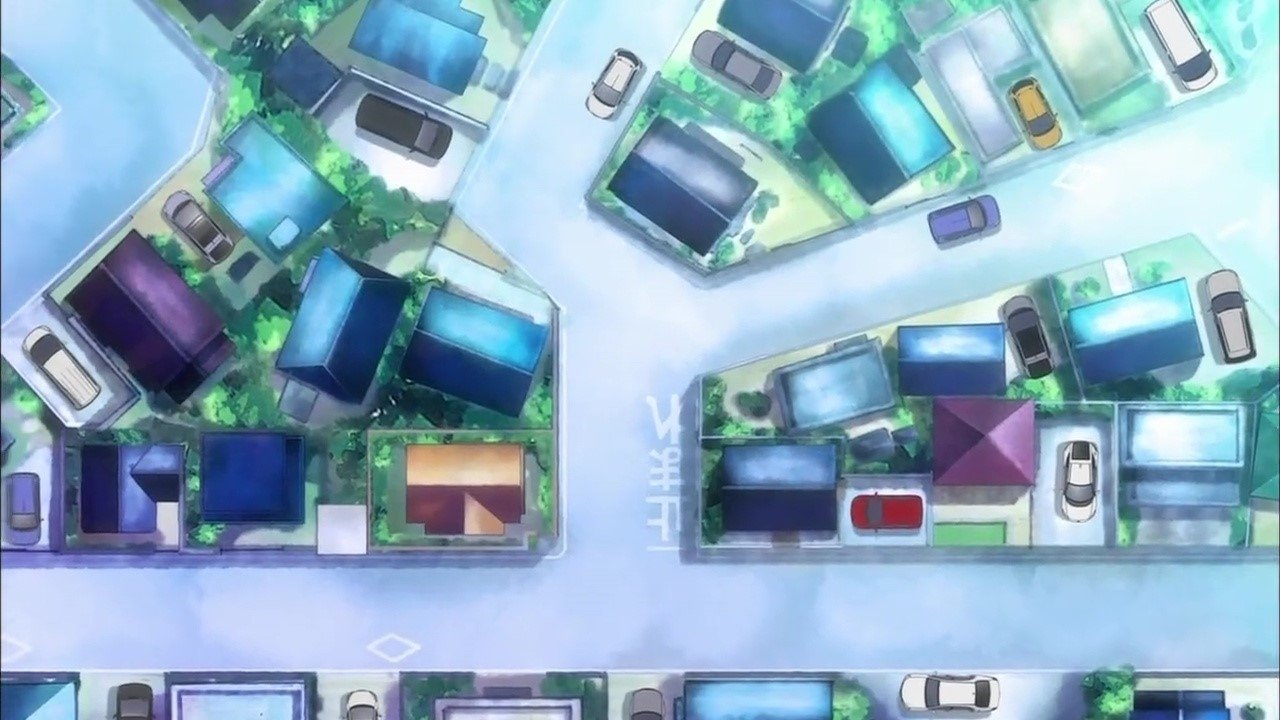
When the exponent is passed to infinity with the sample size, these kernels produce consistent LRV/HAC estimates. They are constructed by exponentiating a mother kernel (a conventional lag kernel that is smooth at the origin) and they can be used without truncation or bandwidth parameters. The kernels are called steep origin kernels and are related to a class of sharp origin kernels explored by the authors (2003) in other work. A new class of kernel estimates is proposed for long run variance (LRV) and heteroskedastic autocorrelation consistent (HAC) estimation.
